-
Notifications
You must be signed in to change notification settings - Fork 388
Commit
This commit does not belong to any branch on this repository, and may belong to a fork outside of the repository.
- Loading branch information
Showing
3 changed files
with
424 additions
and
1 deletion.
There are no files selected for viewing
This file contains bidirectional Unicode text that may be interpreted or compiled differently than what appears below. To review, open the file in an editor that reveals hidden Unicode characters.
Learn more about bidirectional Unicode characters
Original file line number | Diff line number | Diff line change |
---|---|---|
@@ -0,0 +1,311 @@ | ||
--- | ||
title: Get data out of Rerun | ||
order: 700 | ||
--- | ||
|
||
TODO: intro | ||
|
||
## Create some data | ||
|
||
For this tutorial, we use the [face tracking example](https://rerun.io/examples/video-image/face_tracking). | ||
|
||
### Installation | ||
|
||
See the [face tracking installation instruction](https://rerun.io/examples/video-image/face_tracking#run-the-code) | ||
|
||
|
||
### Acquiring data | ||
|
||
Run the example and open/close the mouth. This creates an interesting signal: `/blendshapes/0/jawOpen`. | ||
|
||
Bonus: temporarily hide the camera to temporarily [`Clear`](../reference/types/archetypes/clear.md) the signal. | ||
|
||
TODO: | ||
- add video screenshot of rerun | ||
- add a live viewer pointed at a saved RRD | ||
|
||
|
||
### Display the data of interest | ||
|
||
- Setup a time series view of the signal | ||
- Setup a dataframe view of the signal | ||
- Explore the data => a threshold at 0.15 should do the trick | ||
|
||
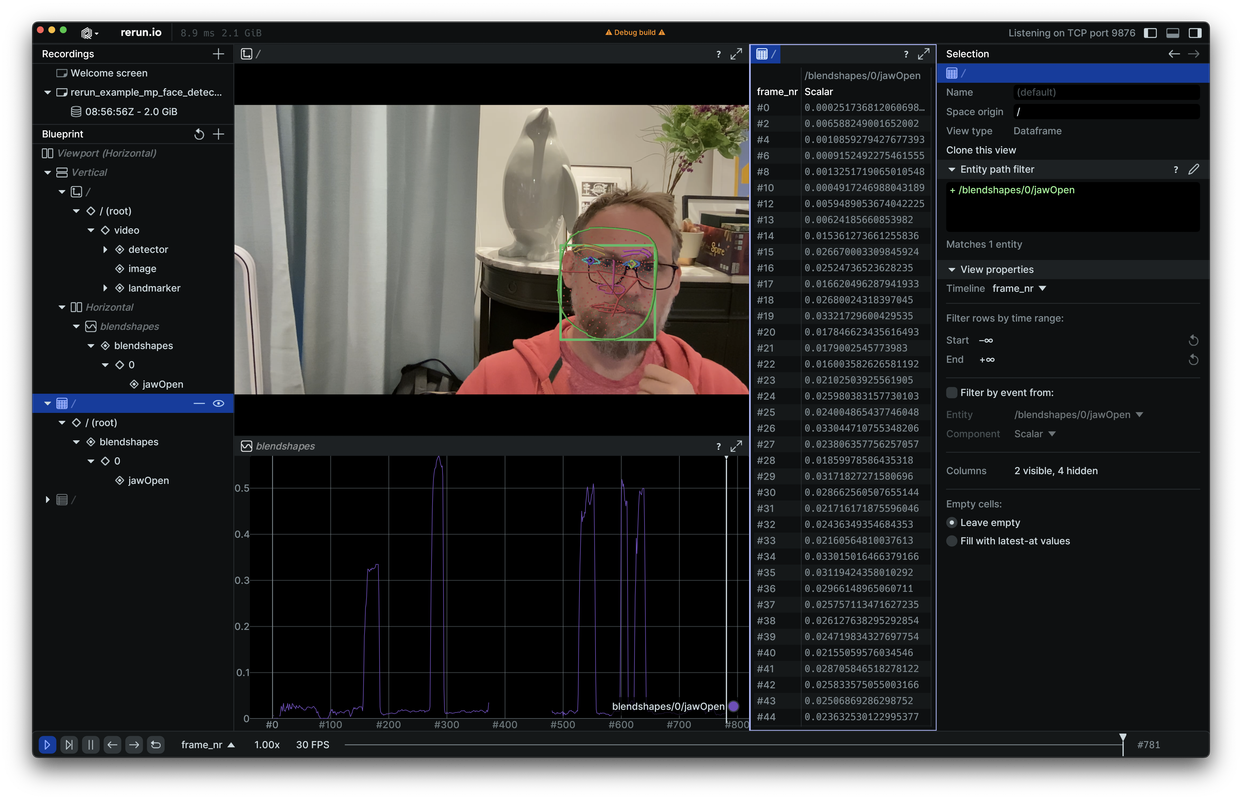 | ||
|
||
TODO: proper screenshot | ||
|
||
|
||
|
||
|
||
|
||
## Analyze the data with Pandas | ||
|
||
|
||
### Load the recording | ||
|
||
- Save the rrd from the viewer. | ||
- In a new python script, load the RRD: | ||
|
||
```python | ||
import rerun as rr | ||
|
||
|
||
def main(): | ||
# TODO: loading directly from the viewer would be sweet | ||
recording = rr.dataframe.load_recording("face_tracking.rrd") | ||
|
||
``` | ||
|
||
### Extract data | ||
|
||
- Copy-as-code from the dataframe view and past the function in the code | ||
- Flatten the `Scalar` column | ||
|
||
TODO: this step is annoying, either smooth it away somehow, or link to some material that explains why data is nested in inner arrays | ||
|
||
|
||
```python | ||
import rerun as rr | ||
import pandas as pd | ||
|
||
SIGNAL_COLUMN = "/blendshapes/0/jawOpen:Scalar" | ||
|
||
# copied from the dataframe view | ||
def export_to_pandas(recording: rr.dataframe.Recording) -> pd.DataFrame: | ||
return ( | ||
recording.view(index="frame_nr", contents="/blendshapes/0/jawOpen") | ||
.select( | ||
rr.dataframe.IndexColumnSelector("frame_nr"), | ||
rr.dataframe.ComponentColumnSelector( | ||
"/blendshapes/0/jawOpen", | ||
"rerun.components.Scalar", | ||
), | ||
) | ||
.read_pandas() | ||
) | ||
|
||
def load_mouth_open_data(recording: rr.dataframe.Recording) -> pd.DataFrame: | ||
df = export_to_pandas(recording) | ||
# TODO: how can we do that better? | ||
df[SIGNAL_COLUMN] = df[SIGNAL_COLUMN].values.flatten() | ||
# df[SIGNAL_COLUMN] = df[SIGNAL_COLUMN].apply(lambda x: x[0] if x is not None else x) # alternative to handle null (slower?) | ||
|
||
return df | ||
|
||
|
||
def main(): | ||
# Load the recording | ||
# TODO: loading directly from the viewer would be sweet | ||
recording = rr.dataframe.load_recording("face_tracking.rrd") | ||
|
||
# Extract the data | ||
df = load_mouth_open_data(recording) | ||
``` | ||
|
||
|
||
### Analyze the data | ||
|
||
- Compute a "mouth open" signal using a threshold | ||
- Extract phase transition between mouth closed -> mouth open | ||
|
||
|
||
```python | ||
import numpy as np | ||
import numpy.typing as npt | ||
import pandas as pd | ||
import rerun as rr | ||
|
||
SIGNAL_COLUMN = "/blendshapes/0/jawOpen:Scalar" | ||
|
||
def export_to_pandas(recording: rr.dataframe.Recording) -> pd.DataFrame: | ||
pass # see above | ||
|
||
|
||
def load_mouth_open_data(recording: rr.dataframe.Recording) -> pd.DataFrame: | ||
pass # see above | ||
|
||
|
||
def analyze_data(df: pd.DataFrame) -> (npt.NDArray, npt.NDArray): | ||
# compute the mouth open state | ||
df["mouth_open"] = (df[SIGNAL_COLUMN] > 0.15).astype(int) | ||
|
||
# find the state transitions | ||
diff = np.diff(df["mouth_open"], prepend=df["mouth_open"].iloc[0]) | ||
open_mouth_frames = df["frame_nr"][diff == 1].values | ||
closed_mouth_frames = df["frame_nr"][diff == -1].values | ||
|
||
# add the initial state | ||
if df["mouth_open"].iloc[0] == 1: | ||
open_mouth_frames = np.concatenate([[0], open_mouth_frames]) | ||
else: | ||
closed_mouth_frames = np.concatenate([[0], closed_mouth_frames]) | ||
|
||
return open_mouth_frames, closed_mouth_frames | ||
|
||
def main(): | ||
# Load the recording | ||
# TODO: loading directly from the viewer would be sweet | ||
recording = rr.dataframe.load_recording("face_tracking.rrd") | ||
|
||
# Extract the data | ||
df = load_mouth_open_data(recording) | ||
|
||
# Process the data | ||
open_mouth_frames, closed_mouth_frames = analyze_data(df) | ||
``` | ||
|
||
|
||
### Plot the data | ||
|
||
```python | ||
import numpy as np | ||
import numpy.typing as npt | ||
import pandas as pd | ||
import rerun as rr | ||
import matplotlib.pyplot as plt | ||
|
||
SIGNAL_COLUMN = "/blendshapes/0/jawOpen:Scalar" | ||
|
||
def export_to_pandas(recording: rr.dataframe.Recording) -> pd.DataFrame: | ||
pass # see above | ||
|
||
|
||
def load_mouth_open_data(recording: rr.dataframe.Recording) -> pd.DataFrame: | ||
pass # see above | ||
|
||
|
||
def analyze_data(df: pd.DataFrame) -> (npt.NDArray, npt.NDArray): | ||
pass # see above | ||
|
||
def plot_analysis(df: pd.DataFrame, open_mouth_frames: npt.NDArray, closed_mouth_frames: npt.NDArray) -> None: | ||
# TODO: bonus points for splitting the series on `frame_nr` discontinuities | ||
|
||
plt.plot(df["frame_nr"], df[SIGNAL_COLUMN], df["frame_nr"], df["mouth_open"]) | ||
plt.plot(open_mouth_frames, np.ones_like(open_mouth_frames), "ro", label="start smiling") | ||
plt.plot(closed_mouth_frames, np.zeros_like(closed_mouth_frames), "go", label="stop smiling") | ||
plt.show() | ||
|
||
|
||
def main(): | ||
# Load the recording | ||
# TODO: loading directly from the viewer would be sweet | ||
recording = rr.dataframe.load_recording("face_tracking.rrd") | ||
|
||
# Extract the data | ||
df = load_mouth_open_data(recording) | ||
|
||
# Process the data | ||
open_mouth_frames, closed_mouth_frames = analyze_data(df) | ||
|
||
# Plot the data | ||
plot_analysis(df, open_mouth_frames, closed_mouth_frames) | ||
``` | ||
|
||
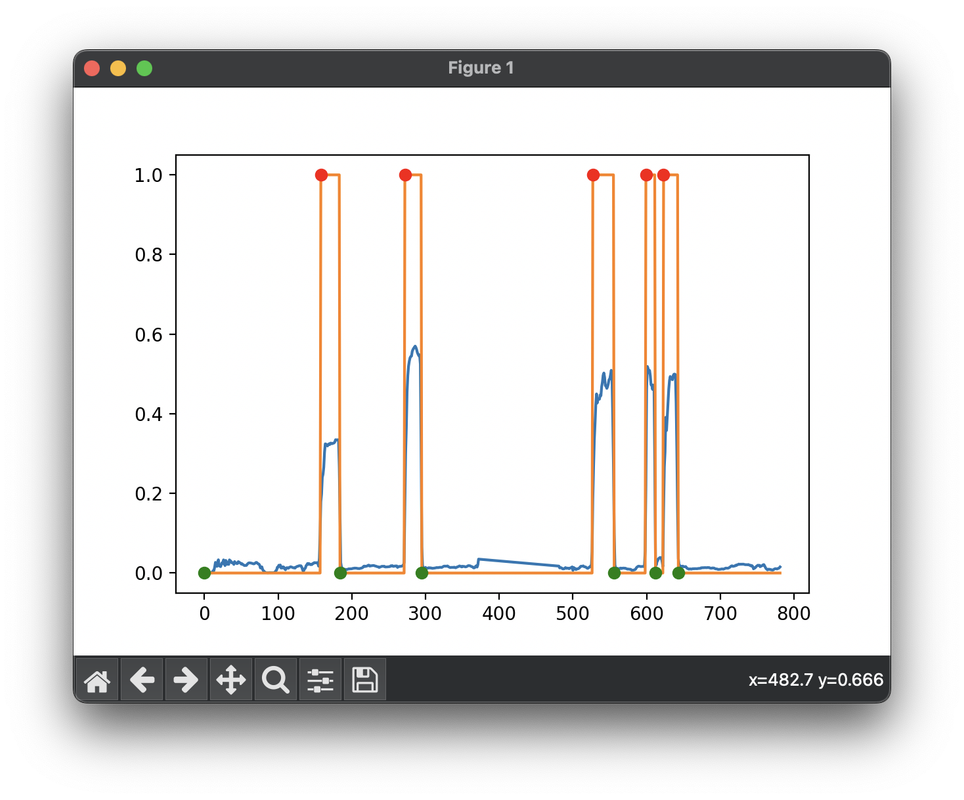 | ||
|
||
TODO: | ||
- improve the plot with legends, etc | ||
- bonus: split the series when there are `Clear`s | ||
|
||
|
||
### Log analysis back to the viewer | ||
|
||
We log the following: | ||
- mouth open state as a bit red dot over the camera view | ||
- mouth state transitions in a `TextLog` view | ||
- mouth state signal as a scalar | ||
|
||
|
||
|
||
```python | ||
import numpy as np | ||
import numpy.typing as npt | ||
import pandas as pd | ||
import rerun as rr | ||
import matplotlib.pyplot as plt | ||
|
||
SIGNAL_COLUMN = "/blendshapes/0/jawOpen:Scalar" | ||
|
||
def export_to_pandas(recording: rr.dataframe.Recording) -> pd.DataFrame: | ||
pass # see above | ||
|
||
def load_mouth_open_data(recording: rr.dataframe.Recording) -> pd.DataFrame: | ||
pass # see above | ||
|
||
def analyze_data(df: pd.DataFrame) -> (npt.NDArray, npt.NDArray): | ||
pass # see above | ||
|
||
def plot_analysis(df: pd.DataFrame, open_mouth_frames: npt.NDArray, closed_mouth_frames: npt.NDArray) -> None: | ||
pass # see above | ||
|
||
def log_analysis(df: pd.DataFrame, open_mouth_frames: npt.NDArray, closed_mouth_frames: npt.NDArray) -> None: | ||
# log state transitions as a red dot showing on top the video feed | ||
for frame_nr in open_mouth_frames: | ||
rr.set_time_sequence("frame_nr", frame_nr) | ||
rr.log("/mouth_open/indicator", rr.Points2D([100, 100], radii=20, colors=[255, 0, 0])) | ||
for frame_nr in closed_mouth_frames: | ||
rr.set_time_sequence("frame_nr", frame_nr) | ||
rr.log("/mouth_open/indicator", rr.Clear(recursive=False)) | ||
|
||
# log state transitions to a TextLog view | ||
for frame_nr in open_mouth_frames: | ||
rr.set_time_sequence("frame_nr", frame_nr) | ||
rr.log("/mouth_open/state", rr.TextLog(f"mouth opened")) | ||
for frame_nr in closed_mouth_frames: | ||
rr.set_time_sequence("frame_nr", frame_nr) | ||
rr.log("/mouth_open/state", rr.TextLog(f"mouth closed")) | ||
|
||
# log the mouth open signal as a scalar | ||
rr.send_columns( | ||
"/mouth_open/values", | ||
times=[rr.TimeSequenceColumn("frame_nr", df["frame_nr"])], | ||
components=[ | ||
rr.components.ScalarBatch(df["mouth_open"].values), | ||
], | ||
) | ||
|
||
def main(): | ||
# Load the recording | ||
# TODO: loading directly from the viewer would be sweet | ||
recording = rr.dataframe.load_recording("face_tracking.rrd") | ||
|
||
# Extract the data | ||
df = load_mouth_open_data(recording) | ||
|
||
# Process the data | ||
open_mouth_frames, closed_mouth_frames = analyze_data(df) | ||
|
||
# Log the analysis results | ||
# TODO: avoid having to copy/paste the recording ID | ||
rr.init("rerun_example_mp_face_detection", recording_id="73a8b473-0711-4b5a-b452-6e79de835299") | ||
rr.connect() | ||
log_analysis(df, open_mouth_frames, closed_mouth_frames) | ||
|
||
# Plot the data | ||
plot_analysis(df, open_mouth_frames, closed_mouth_frames) | ||
``` | ||
|
||
|
||
|
||
### Setup blueprint | ||
|
||
- Add the red dot to the camera view | ||
- Dataframe/timeseries views with both the original and the analyzed signals | ||
- Text log view with the state transitions | ||
|
||
```python | ||
# TODO: soon(tm) | ||
``` | ||
|
||
TODO: screenshot | ||
|
||
|
||
### Complete script | ||
|
||
TODO: | ||
- screenshot | ||
- live viewer pointed at a recording which contains the analysis results | ||
- figure out how to make that maintainable :/ | ||
|
||
snippet: tutorials/data_out_pandas |
This file contains bidirectional Unicode text that may be interpreted or compiled differently than what appears below. To review, open the file in an editor that reveals hidden Unicode characters.
Learn more about bidirectional Unicode characters
Oops, something went wrong.