-
Notifications
You must be signed in to change notification settings - Fork 14
New issue
Have a question about this project? Sign up for a free GitHub account to open an issue and contact its maintainers and the community.
By clicking “Sign up for GitHub”, you agree to our terms of service and privacy statement. We’ll occasionally send you account related emails.
Already on GitHub? Sign in to your account
2018 ICLR Multi-Task Learning for Document Ranking and Query Suggestion #5
Comments
Hi @ZahraTaherikhonakdar |
|
@ZahraTaherikhonakdar any updates? |
In #10 they improved their previous work by learning dependency between users' queries and clicked in a session. They also compare models in #10 with #5. Here are the results that show the improvement:
|
@hosseinfani, as our today's meeting is canceled I describe the novelty of #10 here. Otherwise, I wanted to explain it in the meeting.
|
@ZahraTaherikhonakdar as requested, your progress update should be available by Thursday midnight. We can discuss it in our next meeting. |
Main Problem:
This paper proposed a multi-task learning framework to learn both document ranking and query suggestion. They used users' search log information like clicked documents and sequence of initial queries in one session. They believed that these two tasks have a positive effect on each other and proved it by comparing the performance of their proposed method with the previous document ranking and query suggestion methods.
Input-Output:
phase:
Input: input query+sequence of query in a session(from search log)+candidate documents(from search log)
Output: predicting documents ranking and query suggestion
Previous Works and their Gaps:
Previous query suggestion and documents ranking methods only consider the sequence of queries for query suggestion or clicked documents for document ranking. This paper claimed that by jointly learning query suggestions and documents ranking the performance would increase. Because search behavior like clicked documents and search intent capturing from a sequence of queries in session could have a positive effect on these two tasks.
Result:
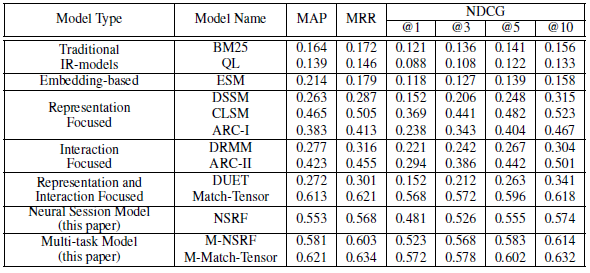
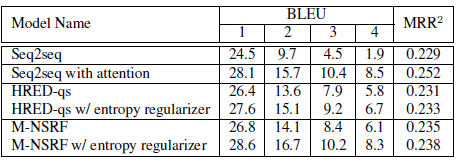
The proposed method significantly improved compared to previous query suggestions and document raking methods.
Document ranking performance :
Query suggestion performance:
Data Set:
They used AOL dataset.
Gap of this work:
This paper used shallow information for training the model. More useful information can be used in training the model to capture users interest and intent in web search and rank documents based on user's behavior. Social information along with search logs can be useful to understand users' intent and improve the qury suggestion and documents ranking tasks.
Code:
https://github.com/wasiahmad/context_attentive_ir
The text was updated successfully, but these errors were encountered: