You signed in with another tab or window. Reload to refresh your session.You signed out in another tab or window. Reload to refresh your session.You switched accounts on another tab or window. Reload to refresh your session.Dismiss alert
Thanks for your great work! I am using your provided model to evaluate single image and find that the visualization is not so good? How could I get better results?
Images are shown here:
Codes are shown below:
Thanks for your great work! I am using your provided model to evaluate single image and find that the visualization is not so good? How could I get better results?
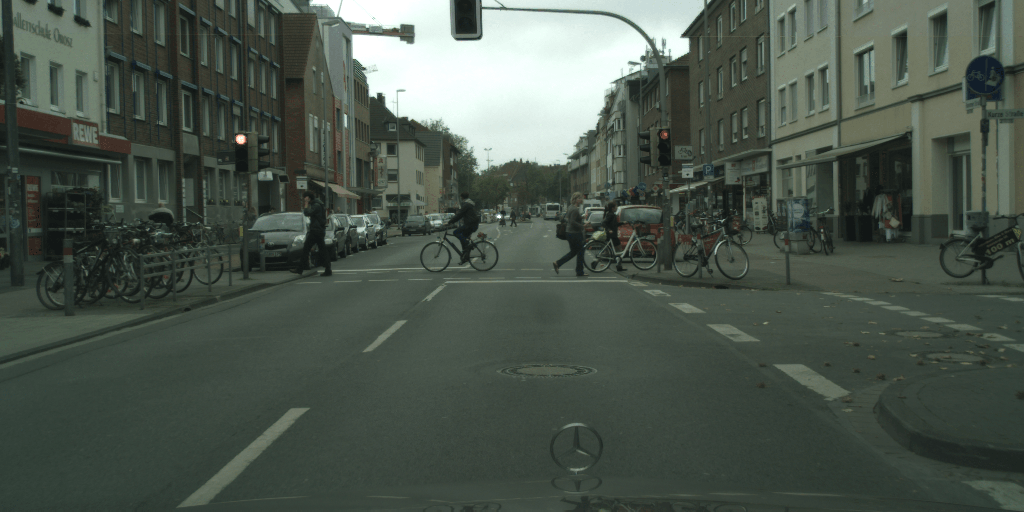
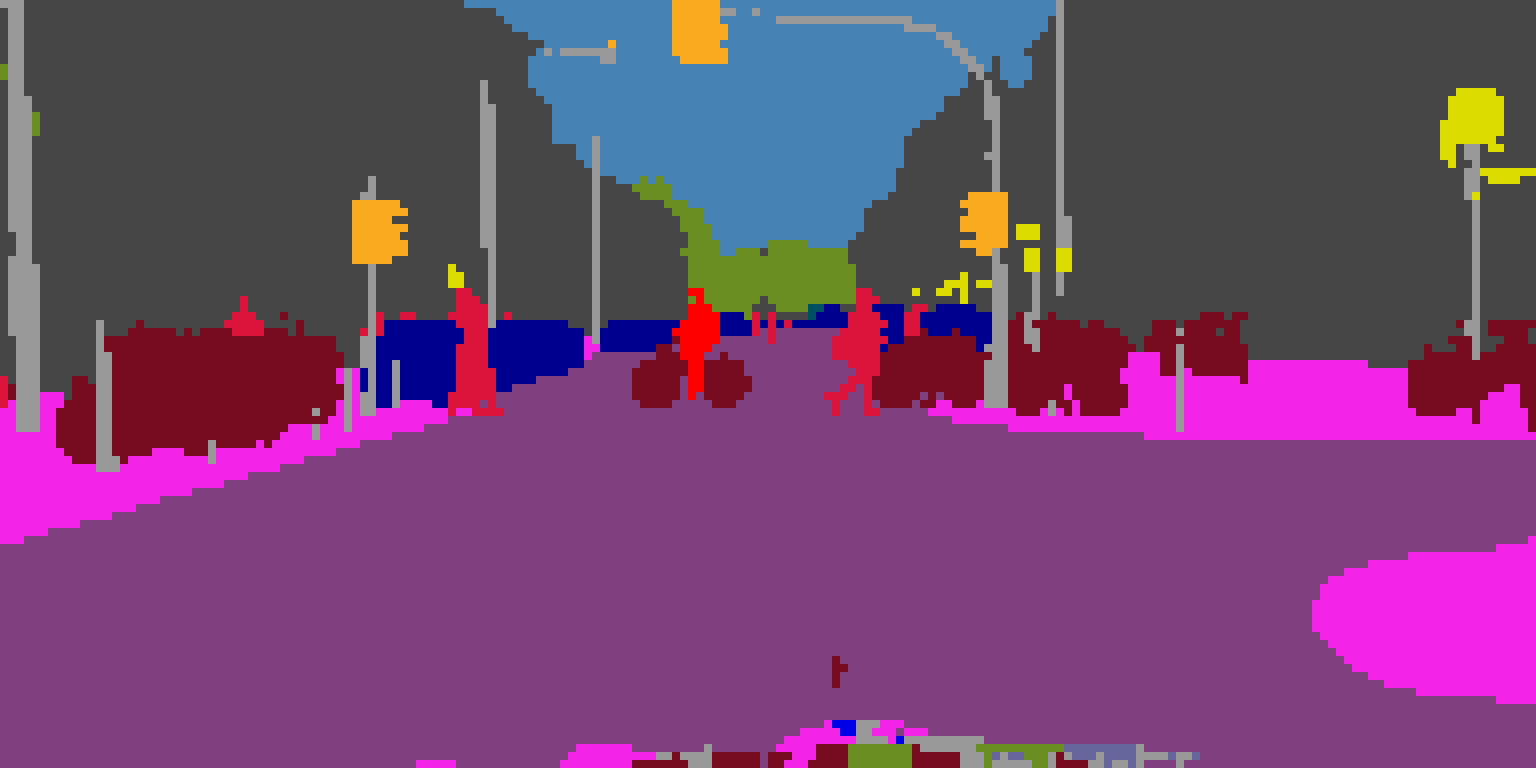
Images are shown here:
Codes are shown below:
code
The text was updated successfully, but these errors were encountered: